1.2 Understanding the Language of Variation
- StatisticaHub
- Dec 8, 2024
- 3 min read
Updated: Dec 9, 2024
AP Statistics: Exploring one variable data
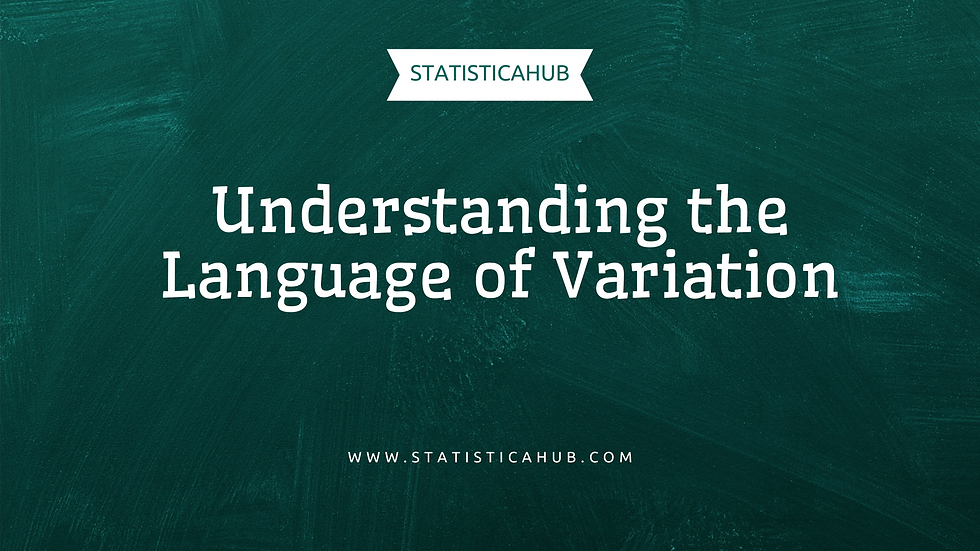
Before diving into the world of statistics, it is crucial to understand the fundamental building blocks: data and variables. The term data (plural) refers to information collected about individuals or units, capturing their characteristics, also known as variables. The values that variables take are collectively referred to as data. Broadly, variables can be classified into two types: categorical and quantitative, and the corresponding data is categorized accordingly.
Categorical Variables:A variable is considered categorical when it assumes values that represent attributes or categories. For instance, car colors, names of countries, or types of cuisines are examples of categorical variables. These values, such as car colors ranging from white to black, are often grouped for comparative analysis.
Quantitative Variables:Quantitative variables, on the other hand, represent measurable characteristics expressed in numerical form. Examples include the price of a product, the age of individuals, or the number of items sold. Quantitative variables are further classified into:
Discrete Variables: Values that are countable and distinct (e.g., the number of children in a household).
Continuous Variables: Values that can take any value within a range (e.g., weight, height).
Measurement Levels of Variables
Variables can also be measured at different levels of granularity, each influencing the type of statistical analysis applicable:
Nominal Level: Categorical data without any inherent order (e.g., hair color, gender).
Ordinal Level: Categorical data with a meaningful order or ranking (e.g., customer satisfaction levels).
Interval Level: Quantitative data with meaningful differences but no true zero (e.g., temperature in Celsius).
Ratio Level: Quantitative data with meaningful differences and a true zero (e.g., weight, income).
Importance of Understanding Variables
Variables inherently change across individuals, introducing variability in data. For instance, responses to the same question from different individuals may vary widely. By leveraging statistical tools, this variability can be analyzed to uncover relationships and patterns, making statistics a fascinating and insightful field.
Categorical vs. Quantitative Variables
To delve deeper, let us explore the distinction between categorical and quantitative variables:
Categorical Variables
These variables classify individuals into groups or categories and cannot be measured numerically. Examples include:
Gender
Marital status
Political affiliation
Eye color
Quantitative Variables
These variables are measurable and can be expressed numerically. They may be:
Continuous: Such as height, weight, or distance traveled.
Discrete: Such as the number of siblings or hospital visits.
Correctly identifying variable types is essential for selecting appropriate statistical techniques. For example, t-tests are typically applied to continuous quantitative data, while chi-square tests analyze categorical data.
Case Study: Transportation Safety
Let’s consider a practical example to illustrate these concepts. The table below provides data on job-related injuries in various transportation industries in 1998:
Industry | Number of Injuries |
Railroad | 4,520 |
Intercity Bus | 5,100 |
Subway | 6,850 |
Trucking | 7,144 |
Airline | 9,950 |
Variables Identified:
Type of Industry: Categorical variable (nominal).
Number of Injuries: Quantitative variable (discrete).
Level of Measurement:
The type of industry is measured at the nominal level.
The number of injuries is measured at the ratio level due to the presence of a meaningful zero.
Insights and Analysis:
The railroad industry has the fewest injuries, while airlines report the highest. However, the data may not fully account for factors such as the size of the workforce in each industry.
This example demonstrates the importance of contextualizing data to derive meaningful insights and to understand the relationships between variables.
Key Takeaways
Individuals: The subjects or units from which data are collected.
Variable: A characteristic that varies across individuals.
Data: The values that variables take.
Categorical Variables: Attributes or categories (qualitative).
Quantitative Variables: Measurable characteristics (numerical).
By carefully organizing and interpreting variables, statisticians can uncover relationships and trends, ultimately enhancing decision-making and understanding of the world around us.
Commentaires